An increasing number of frameworks describe the possible contents of a K–12 artificial intelligence (AI) curriculum and suggest possible learning activities (for example, see the UNESCO competency framework for students, 2024). In our March seminar, Lukas Höper and Carsten Schulte from the Department of Computing Education at Paderborn University in Germany shared with us a unit of work they’ve developed that could inform such a curriculum. At its core, the unit enhances young people’s awareness of how their personal data is used in the data-driven technologies that form part of their everyday lives.
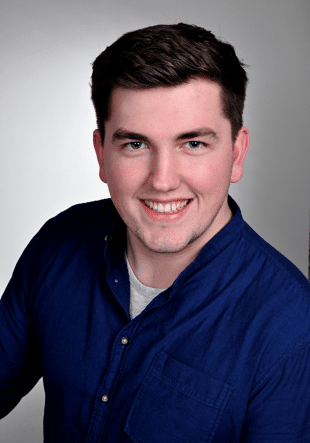
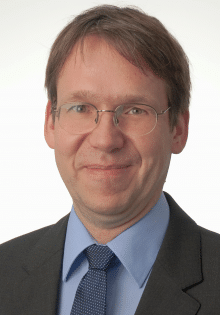
Lukas Höper and Carsten Schulte are part of a larger team who are investigating how to teach school students about data science and Big Data.
Carsten explained that Germany’s informatics (computing) curriculum includes a competency area known as Informatics, People and Society (IPS), which explores the interrelationships between technology, individuals, and society, and how computation influences and is influenced by social, ethical, and cultural factors. However, research has suggested that teachers face several problems in delivering this topic, including:
- Lack of subject knowledge
- Lack of teaching material
- Lack of integration with other topics in informatics lessons
- A perception that IPS is the responsibility of other subjects
Some of the findings of that 2007 research were mirrored in a more recent local study in 2025, which found that although there have been some gains in subject knowledge in the interval period, the problems of a lack of teaching material and integration with other computer science (CS) topics persist, with IPS increasingly perceived as the responsibility of the informatics subject area alone. Despite this, within the informatics curriculum, IPS is often the first topic to be dropped when educators face time constraints — and concerns with what and how to assess the topic remain.
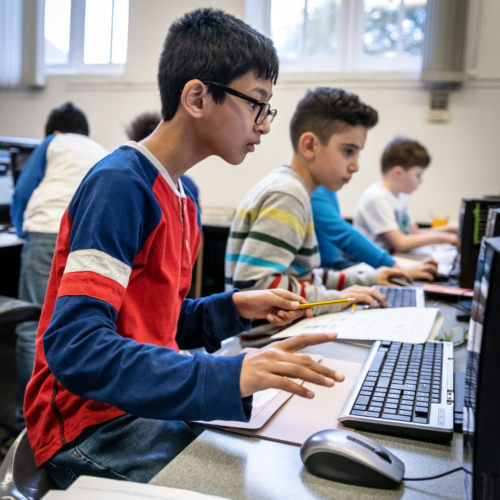
In this context, and as part of a larger, longitudinal project to promote data science teaching in schools called ProDaBi, Carsten and Lukas have been developing, implementing, and evaluating concepts and materials on the topics of data science and AI. Lukas explained the importance of students developing data awareness in the context of the digital systems they use in their everyday lives, such as search engines, streaming services, social media apps, digital assistants, and chatbots, and emphasised the difference between being a user of these systems and a data-aware user. Using the example of image recognition and ‘I am not a robot’ Captcha services, Lukas explained how young people need to develop a data-aware perspective of the secondary purposes of the data collected by these (and other) systems, as well as the more obvious, primary purposes.
Lukas went on to illustrate the human interaction system model, which presents a continuum of possible different roles, from the student as the user of digital artefacts to the student as the designer of digital artefacts.
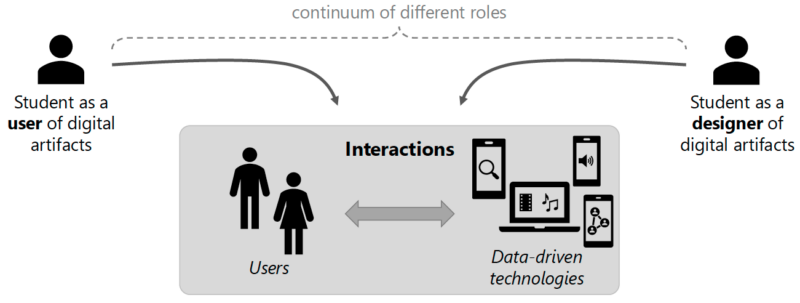
To become data-aware users of digital artefacts, students need to be able to understand and reflect on those digital artefacts. Only then can they proceed to become responsible designers of digital artefacts. However, when surveyed, some students were only moderately interested in engaging with the inner workings of the digital technologies they use in their everyday lives. Many students prefer to use the systems and are less interested in how they process data.
The explanatory model approach in computing education
Lukas explained how students often become more interested in data-driven technologies when learning about them with explanatory models. Such models can foster data awareness, giving students a different perspective of data-driven technologies and helping them become more empowered users of them.
To illustrate, Lukas gave the example of an explanatory model about the role of data in digital systems. Such a model can be used to introduce the idea that data is explicitly and implicitly collected in the interaction between the user and the technology, and used for primary and secondary purposes.
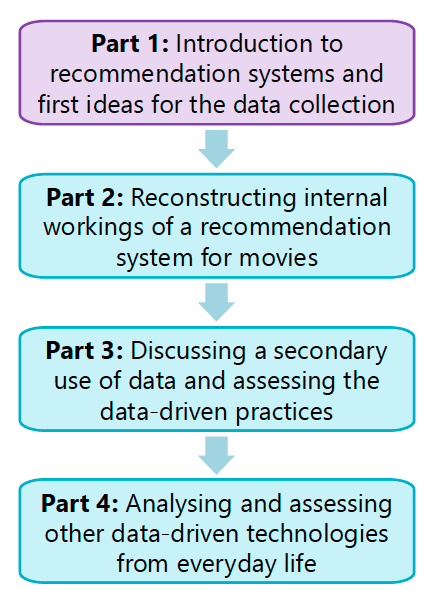
Lukas then introduced two teaching units that were developed for use with middle school children to evaluate the success of the explanatory model approach in computing education. The first unit explores location data collected by mobile phone networks and the second features recommendation systems used by movie streaming services such as Netflix and Amazon Prime.
Taking the second unit as their focus, Lukas and Carsten outlined the four parts of the explanatory model approach:
Part 1
The teaching unit begins by introducing recommendation systems and asking students to think about what a streaming service is, how a personalised start page is constructed, and how personal recommendations might be generated. Students then complete an unplugged activity to simulate the process of making movie recommendations for a peer:
Task 1: Students write down movie recommendations for another student.
Task 2: They then ask each other questions (they collect data).
Task 3: They write down revised movie recommendations.
Task 4: They share and evaluate their recommendations.
Task 5: Together they reflect on which collected data was helpful in this exercise and what kind of data a recommendation system might collect. This reflection introduces the concepts of explicit and implicit data collection.
Part 2
In part 2, students are given a prepared Jupyter Notebook, which allows them to explore a simulation of a recommendation system. Students rate movies and receive personal recommendations. They reconstruct a data model about users, using the idea of collaborative filtering with the k-nearest neighbours algorithm (see Figure 3).
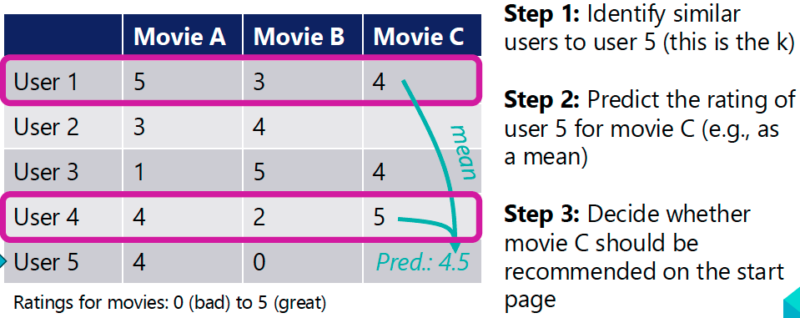
Part 3
In part 3, the concepts of primary and secondary purposes for data collection are introduced. Students discuss examples of secondary purposes such as personalised paywalls for movies that can be purchased, and subscriptions based on the predictions of future behaviour. The discussion includes various topics about individual and societal issues (e.g. filter bubbles, behaviour engineering, information asymmetry, and responsible development of data-driven technologies).
Part 4
Finally, students use the explanatory model as an ‘analytical lens’. They choose other examples from their everyday lives of technologies that implement recommendation systems and analyse these examples, assessing the data practices involved. Students present their results in class and discuss their role in these situations and possible actions they can take to become more empowered, data-aware users.
Uses of explanatory models
Using the explanatory model is one approach to make the Informatics, People and Society strand of the German informatics curriculum more engaging for students, and addresses some of the problems teachers identify with delivering this competency area.
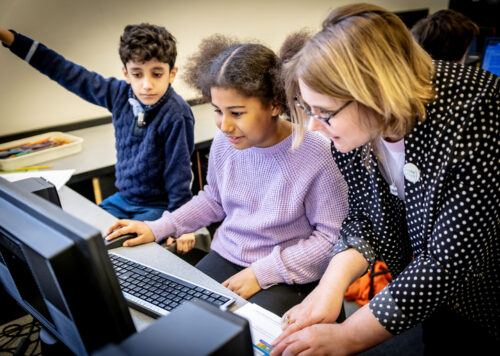
In presenting the idea of the explanatory model, Carsten and Lukas emphasised that the model in use delivers content as well as functioning as a tool to design teaching content. In the example above, we see how the explanatory model introduces the concepts of:
- Explicit and implicit data collection
- Primary and secondary purposes of that data
- Data models
The explanatory model framework can also be used as a focus for academic research in computing education. For example, further research is needed to evaluate if explanatory models are appropriate or ‘correct’ models and to determine the extent to which they are useful in computing education.
In summary, an explanatory model provides a specific perspective on and explanation of particular computing concepts and digital artefacts. In the example given here, the model focuses on the role of data in a recommender system. Explanatory models are representations of concepts, artefacts, and socio-technical systems, but can also serve as tools to support teaching and learning processes and research in computing education.
The teaching units referred to above are published on www.prodabi.de (in German and English).
See the background paper to the seminar, called ‘Learning an explanatory model of data-driven technologies can lead to empowered behaviour: A mixed-methods study in K-12 Computing education’.
You can also view the paper describing the development of the explanatory model approach, called ‘New perspectives on the future of Computing education: Teaching and learning explanatory models’.
Join our next seminar
In our current seminar series, we’re exploring teaching about AI and data science. Join us at our next seminar on Tuesday 13 May at 17:00–18:30 BST to hear Henriikka Vartiainen and Matti Tedre (University of Eastern Finland) discuss how to empower students by teaching them how to develop AI and machine learning (ML) apps without code in the classroom.
To sign up and take part in our research seminars, click below:
You can also view the schedule of our upcoming seminars, and catch up on past seminars on our previous seminars and recordings page.
Website: LINK